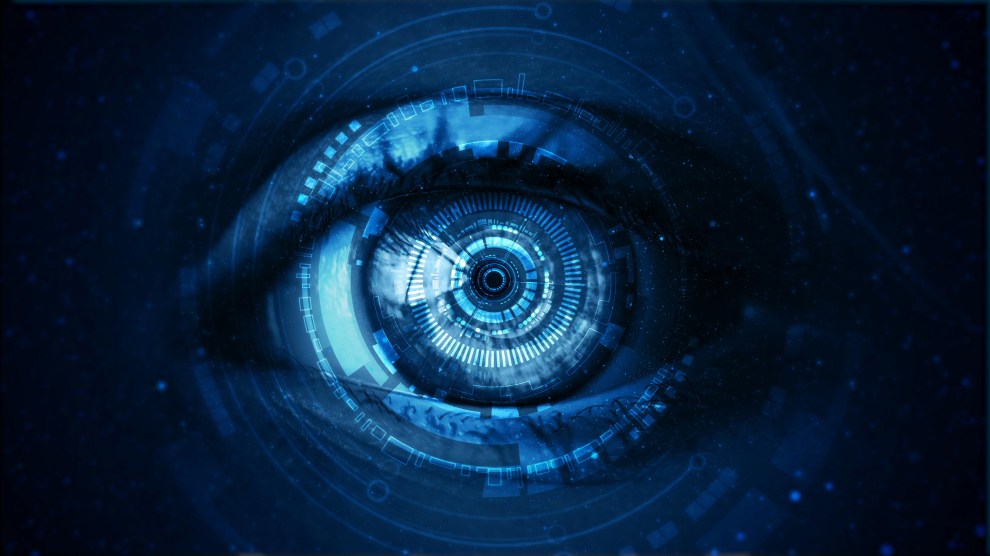
kontekbrothers/Getty Images
This story was originally published by Undark and is reproduced here as part of the Climate Desk collaboration.
Last month, DeepMind, a subsidiary of technology giant Alphabet, set Silicon Valley abuzz when it announced Gato, perhaps the most versatile artificial intelligence model in existence. Billed as a “generalist agent,” Gato can perform over 600 different tasks. It can drive a robot, caption images, identify objects in pictures, and more. It is probably the most advanced AI system on the planet that isn’t dedicated to a singular function. And, to some computing experts, it is evidence that the industry is on the verge of reaching a long-awaited, much-hyped milestone: Artificial General Intelligence.
Unlike ordinary AI, Artificial General Intelligence wouldn’t require giant troves of data to learn a task. Whereas ordinary artificial intelligence has to be pre-trained or programmed to solve a specific set of problems, a general intelligence can learn through intuition and experience.
An AGI would in theory be capable of learning anything that a human can, if given the same access to information. Basically, if you put an AGI on a chip and then put that chip into a robot, the robot could learn to play tennis the same way you or I do: by swinging a racket around and getting a feel for the game. That doesn’t necessarily mean the robot would be sentient or capable of cognition. It wouldn’t have thoughts or emotions, it’d just be really good at learning to do new tasks without human aid.
This would be huge for humanity. Think about everything you could accomplish if you had a machine with the intellectual capacity of a human and the loyalty of a trusted canine companion — a machine that could be physically adapted to suit any purpose. That’s the promise of AGI. It’s C-3PO without the emotions, Lt. Commander Data without the curiosity, and Rosey the Robot without the personality. In the hands of the right developers, it could epitomize the idea of human-centered AI.
But how close, really, is the dream of AGI? And does Gato actually move us closer to it?
For a certain group of scientists and developers (I’ll call this group the “Scaling-Uber-Alles” crowd, adopting a term coined by world-renowned AI expert Gary Marcus) Gato and similar systems based on transformer models of deep learning have already given us the blueprint for building AGI. Essentially, these transformers use humongous databases and billions or trillions of adjustable parameters to predict what will happen next in a sequence.
The Scaling-Uber-Alles crowd, which includes notable names such as OpenAI’s Ilya Sutskever and the University of Texas at Austin’s Alex Dimakis, believes that transformers will inevitably lead to AGI; all that remains is to make them bigger and faster. As Nando de Freitas, a member of the team that created Gato, recently tweeted: “It’s all about scale now! The Game is Over! It’s about making these models bigger, safer, compute efficient, faster at sampling, smarter memory…” De Freitas and company understand that they’ll have to create new algorithms and architectures to support this growth, but they also seem to believe that an AGI will emerge on its own if we keep making models like Gato bigger.
Call me old-fashioned, but when a developer tells me their plan is to wait for an AGI to magically emerge from the miasma of big data like a mudfish from primordial soup, I tend to think they’re skipping a few steps. Apparently, I’m not alone. A host of pundits and scientists, including Marcus, have argued that something fundamental is missing from the grandiose plans to build Gato-like AI into full-fledged generally intelligent machines.
I recently explained my thinking in a trilogy of essays for The Next Web’s Neural vertical, where I’m an editor. In short, a key premise of AGI is that it should be able to obtain its own data. But deep learning models, such as transformer AIs, are little more than machines designed to make inferences relative to the databases that have already been supplied to them. They’re librarians and, as such, they are only as good as their training libraries.
A general intelligence could theoretically figure things out even if it had a tiny database. It would intuit the methodology to accomplish its task based on nothing more than its ability to choose which external data was and wasn’t important, like a human deciding where to place their attention.
Gato is cool and there’s nothing quite like it. But, essentially, it is a clever package that arguably presents the illusion of a general AI through the expert use of big data. Its giant database, for example, probably contains datasets built on the entire contents of websites such as Reddit and Wikipedia. It’s amazing that humans have managed to do so much with simple algorithms just by forcing them to parse more data.
In fact, Gato is such an impressive way to fake general intelligence, it makes me wonder if we might be barking up the wrong tree. Many of the tasks Gato is capable of today were once believed to be something only an AGI could do. It feels like the more we accomplish with regular AI, the harder the challenge of building a general agent appears to be.
For those reasons, I’m skeptical that deep learning alone is the path to AGI. I believe we’ll need more than bigger databases and additional parameters to tweak. We’ll need an entirely new conceptual approach to machine learning.
I do think that humanity will eventually succeed in the quest to build AGI. My best guess is that we will knock on AGI’s door sometime around the early-to-mid 2100s, and that, when we do, we’ll find that it looks quite different from what the scientists at DeepMind are envisioning.
But the beautiful thing about science is that you have to show your work, and, right now, DeepMind is doing just that. It’s got every opportunity to prove me and the other naysayers wrong.
I truly, deeply hope it succeeds.